How We Harness Generative AI’s Established Neural Networks For Your Industry’s Applications
- ridgerun
- Jul 1, 2024
- 4 min read
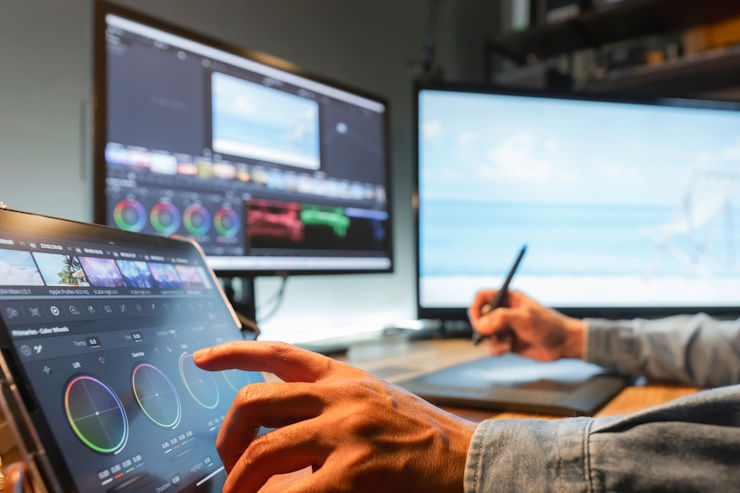
Foundational Models Provide the Baseline For Your Vision - and Our Expertise
Generative AI (or GenAI for short) is a family of deep learning models that can generate content (output) based on the data (input) it is provided. Using specially crafted artificial neural networks, which are named for their similarity to how the human brain processes information, the model receives data, analyzes it against the patterns it learned, using the massive amounts of data it was trained on, and provides a response.
Specific applications, such as ChatGPT, allow the user to invoke the model by entering a request to receive feedback (e.g., “Give me a list of grocery items needed to make homemade pasta”). DALL-E, another well-known application that uses generative AI, can create an image from a description provided by the user (e.g., “Show me a picture of authentic-looking pasta from Italy”). These requests, or input descriptions, are popularly known as the prompt.
Most people who engage with technology regularly have encountered the following use cases for GenAI. The many GenAI applications we use today demonstrate just how much deep learning is changing our lifestyles at work and home:
Graphics or image generation
Design creation
Email and memo writing
Translation
Proposal writing
Summarization
Voice generation
Much of the software we use today includes AI functionality to provide easier access to content, whether we’re designing invitations, requiring a summary of a length report for work, or brainstorming a workout plan.
Generative AI’s Most Effective Neural Networks
When a user provides a prompt to a generative AI model, it passes through thousands of layers of the neural networks. Each of these layers consists of nodes, which are the neural processing units for the data they receive. Nodes contain weights, which are the trainable parameters of a neural network.
These nodes are interconnected throughout the network’s many layers, and perform several mathematical operations, allowing the model to make complex connections between vast amounts of data and determine the most accurate and effective answer to the prompt.
The most commonly used neural networks for generative AI applications are transformer neural networks and diffusion neural networks.
Architectures based on transformers are highly effective at processing language to effectively contextualize data and prioritize the importance of the different words in the prompt to correctly respond to the user.
Architectures based on diffusion learn to remove noise from an image. The amount of noise added to train images increases until the image becomes unrecognizable. Then, the model can generate image content from pure noise. This is particularly useful for image creation and photo editing. The model can create a requested image based on its training with similar images (e.g., “Make me an image of a cat eating homemade pasta”) or create noise around a specific aspect of an image to denoise it with a desired result (e.g., “Remove the pasta sauce stain from my t-shirt in this picture”).
How We Leverage Neural Network Training For Gen AI Use Cases
The work of creating artificial neural networks and training generative AI is typically left in the hands of tech corporations with significant resources, such as NVIDIA. As an NVIDIA preferred partner, RidgeRun.ai is prepared to deliver expertise that advances their work. Our role begins when our clients seek to leverage generative AI models for their specific use cases.
As an illustration of our work, our synthetic haze tool demonstrates the part we play in advancing AI today. RidgeRun.ai was tasked with creating generative AI-based artifacts of hazy or murky conditions for clients who desire clearer video streams.
Autonomous robots, such as drones or underwater vehicles, depend upon exceptional visual clarity to fulfill their missions. Bird’s eye photography, 3D mapping, surveillance, and disaster evaluation, along with ecological monitoring, oil and gas exploration, and more rely on the ability to function in less-than-ideal environments.
RidgeRun.ai conducted significant research to create a synthetic haze tool, which can be used to train models used for de-hazing, and promises to open additional use cases, such as the removal of smoke, rain, and other clarity-obscuring conditions.
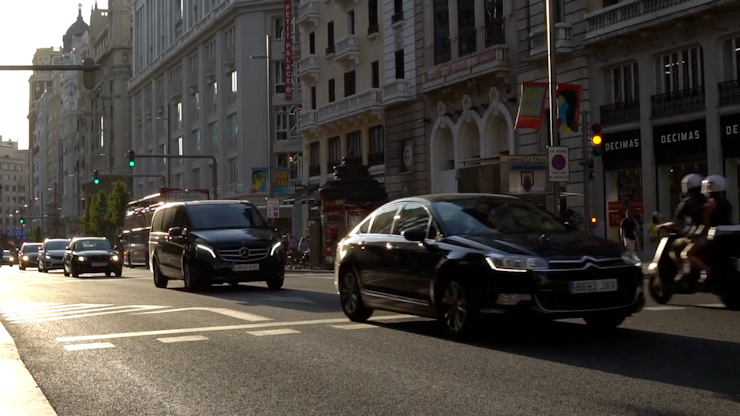

Our commitment to behind-the-scenes, complex data generation plays an important role in supporting critical down-the-line solutions. We deliver the fine-tuning that ensures that our clients’ journey to market is faster and more efficient.
Read more about our work with synthetic haze at our resources hub for developers.
Partner With RidgeRun.ai to Optimize Gen AI Neural Network Models
Much attention has been paid to transformer neural networks use cases, like ChatGPT, and diffusion models, like DALL-E. However, these foundational models and widely popular applications shouldn’t steal the show. It is important to look at them as an opportunity and a blank canvas upon which you can create your groundbreaking deep learning use cases.
RidgeRun.ai’s capabilities for leveraging established models and making improvements and customizations for our customers are virtually limitless. Our comfort and agility with the foundational models, neural networks, and neural processing units that make deep learning possible today allow us to create efficient solutions for our clients. You simply need to share your vision with us, and we can help bring it to life.
Contact RidgeRun.ai today to begin a partnership. With our engineering expertise, your concept for a deep learning product receives the enhancements and optimizations that are vital for real-world deployment.
Comments